Loading...
What can hockey teach us about AI? It turns out, quite a lot!
On this episode of Approximately Correct, we look at how the fast-paced and unpredictable game of hockey makes it a great laboratory to learn about AI teamwork that could mean big benefits in applications like industry and robotics.
Hosts Alona Fyshe and Scott Lilwall are joined by David Radke, Senior Research Scientist with the Chicago Blackhawks and a PhD graduate of the University of Waterloo. David gave a fascinating presentation on his research at last year’s Upper Bound conference.
With NHL playoffs in full swing and this year’s Upper Bound only a week away, including more sessions on AI in sports, we were excited to score an interview with him on how he is using hockey to advance research into multi-agent systems.
"That was kind of the theme of my research," David says, "how teams of agents can learn to work together to learn behavior that is better than anything that humans could encode."
“A decision-making science”
As David points out, a hockey game offers a unique mix of competition and cooperation that makes it an exciting laboratory for studying how individuals work together. He found it the perfect place to explore his fascination for cooperative AI – how individual agents can learn to work together, even when short-term incentives might suggest otherwise.
Statistics have always been a key part of professional sports — one only needs to look at how Moneyball-style analytics have changed the playing field in pro baseball. But David explains that “invasion games” like hockey, where teams have to try to invade their opponent’s side of the ice to score points, offer a completely different opportunity. Since there is a lot more interaction between players in hockey, both friend and foe, it can teach us more about how AI agents might work together.
"In invasion games, it's not really about measuring the execution of players, but it's about measuring the decisions that players make," David says.
“And reinforcement learning is a decision-making science.”
Impact beyond the ice
His research also shines a light on what makes a team truly efficient. And these insights could have a big impact off the ice. Many challenges in hockey mirror those in broader multi-agent AI research: for instance, understanding how agents learn to cooperate, assign credit for good decisions, and develop strategies that balance individual reward and team success. Those answers could offer huge benefits to fields from industrial robotics to self-driving vehicles to even business strategy — anywhere where cooperation and teamwork are important.
Check out the episode on Apple Podcasts, Spotify, or YouTube. And if you’re interested in learning more about how machine learning is transforming all levels of sports, this year’s Upper Bound conference continues the exploration of how NHL teams are using machine learning to up their game with Meghan Chayka, CEO of Stathletes and frequent TSN commentator. As well as sessions on how basketball is being used along with machine learning, to build tech literacy in students. You can learn more about that on the Upper Bound website.
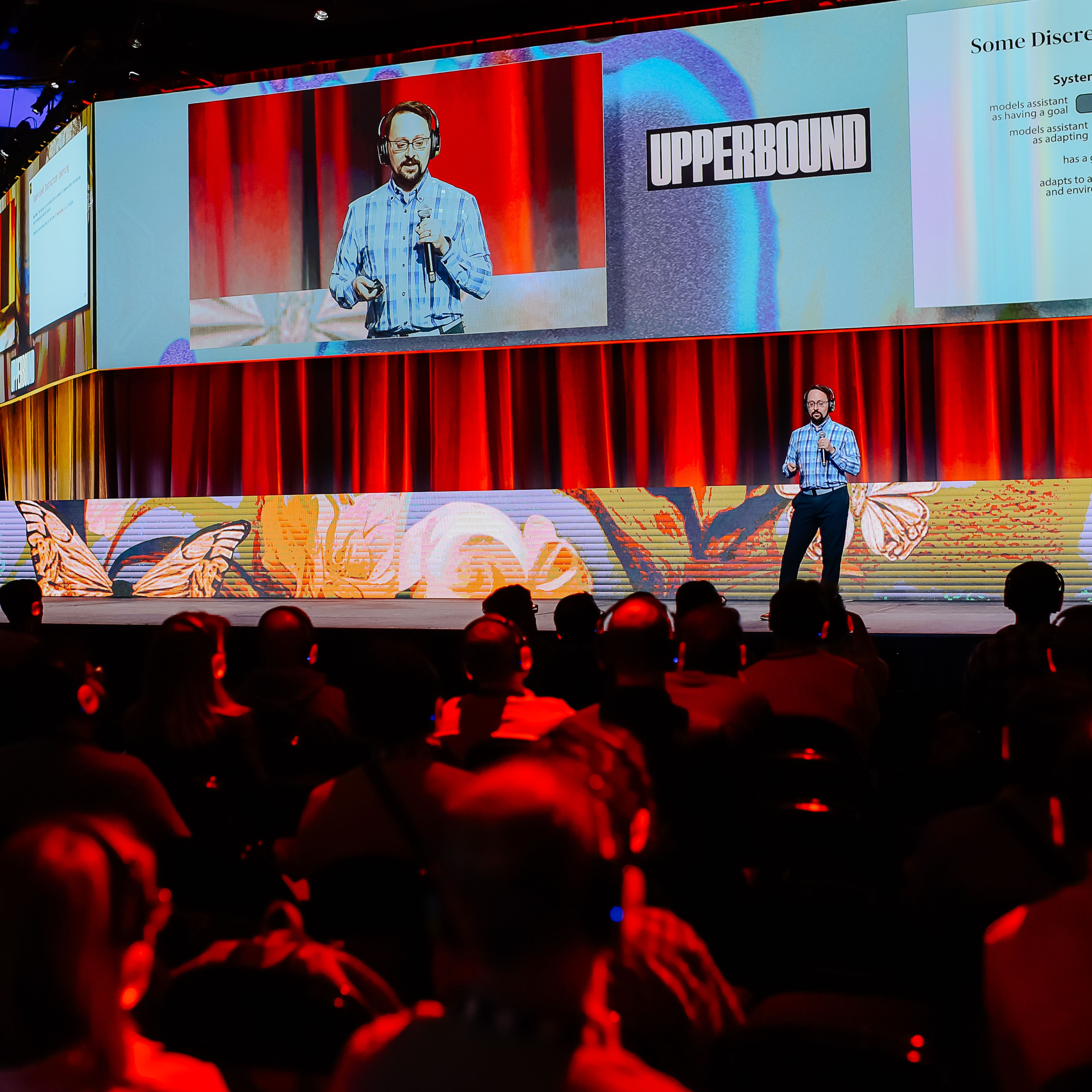
Not Your Average AI Conference
Learn from Leading Minds in AI at Upper Bound
Be one of the thousands of AI professionals, researchers, business leaders, entrepreneurs, investors, and students in Edmonton this spring. Explore new ideas, challenge the status quo, and help shape a positive AI future.